Taming the AI Wild West: The Need for AI Governance
By Debu Chatterjee, Co-founder and CEO, Konfer
As countries and regulatory authorities try to contain AI’s rapid adoption, the software industry may find itself quickly becoming regulated. Such regulations can be anti-competitive if the cost of regulatory compliance is deemed too high, on the one hand. On the other hand, a streamlined approach can lead to reduced costs and scales as the number of assets and regulations increases. I believe, as discussed below, that this rush to regulate is motivated, in part, by the breakdown the U.S. experienced during the administration of the Payroll Protection Program.
Of the $1.2 trillion or so that the U.S. government disbursed during the Payroll Protection Program (PPP), the Small Business Administration (SBA) Office of Inspector General (OIG) published a report that estimates about $200B (yes, billion!) was lost due to fraud. In December, the bipartisan House Select Committee on the Coronavirus Crisis released its report titled “How Fintechs Facilitated Fraud in the Paycheck Protection Program,”1 which advanced the idea that the lack of oversight regarding Finance Technologies (FinTechs) allowed large amounts of fake PPP loans to be dispersed. “While the PPP delivered vital relief to millions of eligible small businesses, at least tens of billions of dollars in PPP funds were likely disbursed to ineligible or fraudulent applicants, often with the involvement of fintechs, causing tremendous harm to taxpayers,” the staff of the House Select Subcommittee on the Coronavirus Crisis wrote in the December report.
“Fintechs are claiming an increasingly large role in the financial industry, from providing application portals to borrowers as they did in the PPP, to housing encrypted transactions on complex networks,” the staff report stated2. “Although fintechs often behave like banks and traditional depository institutions, they are not subject to banking regulations such as the Bank Secrecy Act, which would require them to implement certain processes and structures to ensure the safety and soundness of their operations.”
Many of these fintechs used some form of artificial intelligence (AI) to process credit risk, loan amount decisions, and disbursements. In the rush to provide funds, these tools were allowed to be executed without oversight or governance.
Examples of AI-based decision-making are increasingly coming under scrutiny today—from job board bias in New York City to claims denials in healthcare. While AI is helping improve decision-making for enterprises, it is also becoming imperative to prove that the process of AI-based decision-making is explainable—what assets were used, how, and what was the logic?
Following the financial crash of 2008, when banks and financial institutions were reined in for their wildly unpredictable lending practices, in 2010, the Dodd–Frank Wall Street Reform and Consumer Protection Act was enacted in the US as a response to the crisis to "promote the financial stability of the United States." The Basel III capital and liquidity standards were also adopted by countries around the world.
Today, AI is in a similar situation. Countries and ethics groups are stepping up to announce their AI policies—varying from guidance to detailed requirements. Global corporations are now faced with complexities that could hinder confident AI innovation and adoption.
Questions raised may include:
- How do we know we have any AI working in our organization? (Most software today has some AI in it, and business leaders may be unaware of them).
- Which of our businesses are impacted by which specific AI regulatory mandate?
- What are our risks, and do we know how to manage them?
- Our global footprint could cause our organization to be exposed to multiple agency oversight, regulations, and mandates. How do we keep pace with them?
A comprehensive AI Governance, Risk, and Compliance (GRC) solution will help enterprises accelerate their AI adoption and innovation while being compliant with evolving global regulations. Adapting from a post by Thomson Reuters, an AI GRC solution can help create a playbook that offers:
Future Scanning
Scan and evaluate proposed or pending legislation, rules, enforcement actions, and public comments made by regulators to detect future risks and concerns.
Obligation Libraries and Regulatory Change Management
Monitor current regulatory obligations and notifications while comparing and tracking regulatory changes and notifications to help stay current. This library can be deployed across assets, business functions, and geographies to drive continuous AI Governance.
Policy Management
AI GRC will help map regulations and change management and coordinate it with an organization’s current policies and procedures. It will better detect gaps and necessary policy changes, and it may also suggest risk postures for the updates to fill such gaps.
The advantage of an AI GRC solution is the playbook model—build once and deploy multiple times across multiple businesses and geographies, so that AI risks are continuously monitored and managed for their exposure. A playbook is a guided model to accelerate the development and deployment of any solution—learning from what works and doesn’t work, reducing errors by prescribing a guided model, and adapting to different industries and use cases without having to code from the start.
Additionally, generative AI provides the ability to ask complex questions and receive plain language answers, which could minimize risks and improve communications between senior executives, such as chief technology officers (CTOs), chief information security officers (CISOs), and their organizations’ boards of directors.
AI adoption and governance is not a department, it is the whole company’s responsibility. Bringing developers, managers, and executive leadership to the same level of transparency requires a ground-up view of how AI tools are being used. An AI GRC product can bring it all together and make it seamless to deploy and use.
[1] We are Not the Fraud Police: How Fintechs Facilitated Fraud in the Paycheck Protection Program. Staff Report from the Select Subcommittee on the Coronavirus Crisis, December 2022.
[2] Refer to page 85 in the report.
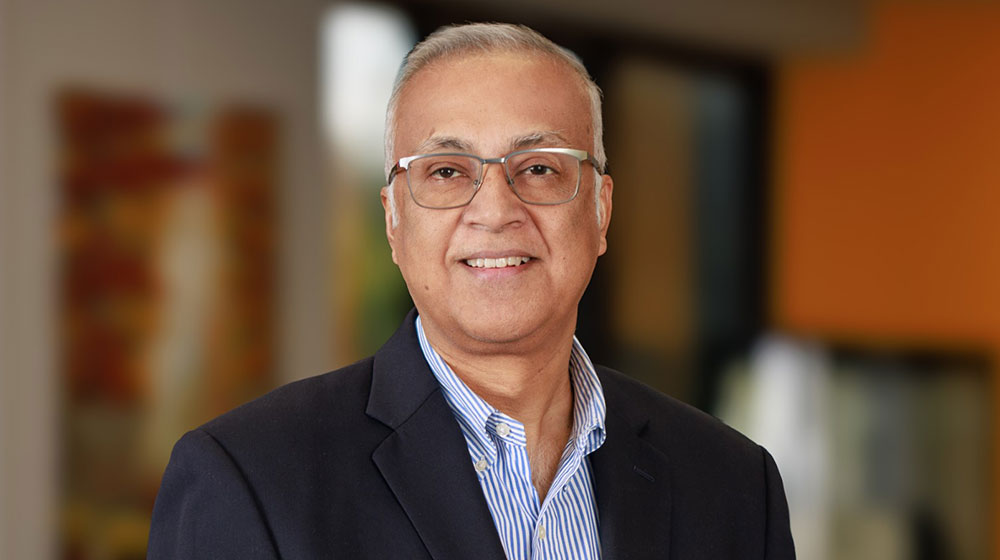
About the author
Debu Chatterjee is the CEO and Co-founder at Konfer, the industry-first AI GRC product that leverages generative AI to help enterprises embrace a Governance By Design AI risk and compliance management program. He was previously the CEO of DxContinuum, which was acquired by ServiceNow, where he subsequently led the AI growth initiatives for the company. Prior to his entrepreneurial ventures, he led technology at FICO, Informatica, and Oracle. He holds an MBA from Wharton, and degrees from UNC Chapel Hill and the Indian Institute of Technology, Kharagpur, India.
By Debu Chatterjee, Co-founder and CEO, Konfer
As countries and regulatory authorities try to contain AI’s rapid adoption, the software industry may find itself quickly becoming regulated. Such regulations can be anti-competitive if the cost of regulatory compliance is deemed too high, on the one hand. On the other hand, a streamlined approach can lead to reduced costs and scales as the number of assets and regulations increases. I believe, as discussed below, that this rush to regulate is motivated, in part, by the breakdown the U.S. experienced during the administration of the Payroll Protection Program.
Of the $1.2 trillion or so that the U.S. government disbursed during the Payroll Protection Program (PPP), the Small Business Administration (SBA) Office of Inspector General (OIG) published a report that estimates about $200B (yes, billion!) was lost due to fraud. In December, the bipartisan House Select Committee on the Coronavirus Crisis released its report titled “How Fintechs Facilitated Fraud in the Paycheck Protection Program,”1 which advanced the idea that the lack of oversight regarding Finance Technologies (FinTechs) allowed large amounts of fake PPP loans to be dispersed. “While the PPP delivered vital relief to millions of eligible small businesses, at least tens of billions of dollars in PPP funds were likely disbursed to ineligible or fraudulent applicants, often with the involvement of fintechs, causing tremendous harm to taxpayers,” the staff of the House Select Subcommittee on the Coronavirus Crisis wrote in the December report.
“Fintechs are claiming an increasingly large role in the financial industry, from providing application portals to borrowers as they did in the PPP, to housing encrypted transactions on complex networks,” the staff report stated2. “Although fintechs often behave like banks and traditional depository institutions, they are not subject to banking regulations such as the Bank Secrecy Act, which would require them to implement certain processes and structures to ensure the safety and soundness of their operations.”
Many of these fintechs used some form of artificial intelligence (AI) to process credit risk, loan amount decisions, and disbursements. In the rush to provide funds, these tools were allowed to be executed without oversight or governance.
Examples of AI-based decision-making are increasingly coming under scrutiny today—from job board bias in New York City to claims denials in healthcare. While AI is helping improve decision-making for enterprises, it is also becoming imperative to prove that the process of AI-based decision-making is explainable—what assets were used, how, and what was the logic?
Following the financial crash of 2008, when banks and financial institutions were reined in for their wildly unpredictable lending practices, in 2010, the Dodd–Frank Wall Street Reform and Consumer Protection Act was enacted in the US as a response to the crisis to "promote the financial stability of the United States." The Basel III capital and liquidity standards were also adopted by countries around the world.
Today, AI is in a similar situation. Countries and ethics groups are stepping up to announce their AI policies—varying from guidance to detailed requirements. Global corporations are now faced with complexities that could hinder confident AI innovation and adoption.
Questions raised may include:
- How do we know we have any AI working in our organization? (Most software today has some AI in it, and business leaders may be unaware of them).
- Which of our businesses are impacted by which specific AI regulatory mandate?
- What are our risks, and do we know how to manage them?
- Our global footprint could cause our organization to be exposed to multiple agency oversight, regulations, and mandates. How do we keep pace with them?
A comprehensive AI Governance, Risk, and Compliance (GRC) solution will help enterprises accelerate their AI adoption and innovation while being compliant with evolving global regulations. Adapting from a post by Thomson Reuters, an AI GRC solution can help create a playbook that offers:
Future Scanning
Scan and evaluate proposed or pending legislation, rules, enforcement actions, and public comments made by regulators to detect future risks and concerns.
Obligation Libraries and Regulatory Change Management
Monitor current regulatory obligations and notifications while comparing and tracking regulatory changes and notifications to help stay current. This library can be deployed across assets, business functions, and geographies to drive continuous AI Governance.
Policy Management
AI GRC will help map regulations and change management and coordinate it with an organization’s current policies and procedures. It will better detect gaps and necessary policy changes, and it may also suggest risk postures for the updates to fill such gaps.
The advantage of an AI GRC solution is the playbook model—build once and deploy multiple times across multiple businesses and geographies, so that AI risks are continuously monitored and managed for their exposure. A playbook is a guided model to accelerate the development and deployment of any solution—learning from what works and doesn’t work, reducing errors by prescribing a guided model, and adapting to different industries and use cases without having to code from the start.
Additionally, generative AI provides the ability to ask complex questions and receive plain language answers, which could minimize risks and improve communications between senior executives, such as chief technology officers (CTOs), chief information security officers (CISOs), and their organizations’ boards of directors.
AI adoption and governance is not a department, it is the whole company’s responsibility. Bringing developers, managers, and executive leadership to the same level of transparency requires a ground-up view of how AI tools are being used. An AI GRC product can bring it all together and make it seamless to deploy and use.
[1] We are Not the Fraud Police: How Fintechs Facilitated Fraud in the Paycheck Protection Program. Staff Report from the Select Subcommittee on the Coronavirus Crisis, December 2022.
[2] Refer to page 85 in the report.