An Initial AI Checklist for the Modern Firm
By Steven Dickerson, Ph.D. and Gaurav Sharma
AI is the new tool1 that will be incorporated into most processes within every firm. This journey will start with several use cases and then scale across the firm in time. The process will create both substantial value and risk. Therefore, senior leadership must ensure their organizations are ready for AI at scale. Specifically, the corporate governance structures and organizational framework of each firm must evolve to incorporate AI usage and be flexible enough to manage the changes to come. Starting with the right foundation will allow the firm to embrace AI throughout this journey. We propose a six-point checklist to assist in the initial structuring and rollout of AI:
Principle-based policy for AI use
The first step is developing a policy for your organization to follow when incorporating AI into business processes. This policy should be based on guiding principles, live within the corporate management system, have continuity with the vision and mission of the firm, and be aligned with the firm’s culture. It will provide a framework for meaningful discussions within the organization to create a path to successful integration of AI. Even if some of these principles seem out of reach at first, it is important to articulate them to clarify your firm’s aspirational focus. This is a significant change in the way teams and people work and, like all changes, will face resistance and best practices will need to be developed to support teams through this process.
The scope of the policy should reflect existing and anticipated AI usages, as well as define what will be out of bounds of the policy. The policy should also identify who “owns” the policy and define how the policy fits into the organization. We suggest including language to mitigate any disparity of approaches across the organization. This common set of principles should guide the organization throughout the lifecycle of AI: design, development, implementation, and use. Special consideration should be given to how the common set of principles applies to the acquisition and use of vendor-provided AI.
Responsible party
The policy’s zones of implementation should initially be focused on the first anticipated use cases and impact areas. It may be prudent, initially, to resist centralizing the ownership until a realistic assessment of short-term value versus long-term effort has been made. Eventually, AI needs to (1) affect a business process and (2) find its way to production for it to add value on a sustained basis. Ultimately, the risk is owned by the business, and since both of the above are business functions, the responsible party should be the business process owner. Also, the additional risk from incorporating AI into the business needs to be incorporated into the overall risk governance processes, in partnership with the risk function.
Communication
The incorporation of AI into existing processes is akin to the incorporation of customer interactions and transactions into the web in the late 1990s. There will be a lot of hyperbole, overassessment of capabilities, apprehension amongst people who think their jobs may be impacted, and underestimation of risk. The key here is to communicate candidly how you are thinking about AI, its role, the rationales for implementing it, and the first use cases. Once the use cases have been identified, seek input from subject matter experts to fine-tune the assumptions of your team to provide the best foundation for subsequent work.
Inventory
Once you have a policy that determines how AI will be integrated into your business processes, it is time to inventory existing and potential uses of AI. Using an open definition of appropriate use cases in this effort is helpful. Starting with a broader definition allows the exclusion of inventoried items rather than duplicating the effort if something is missed because of an overly narrow definition. The definition can always be refined later if it proves too broad. The inventory should include any AI developed in-house or externally, including AI embedded in a product. The inventory should capture general identification, data, methodology, and usage information for each usage. Additional questions about whether it is consistent with the guiding principles of the policy will help. The goal here is to capture the inventory as a first step.
Monitoring
Monitoring is also an essential initial step. We suggest “regularly monitored” as one of the guiding principles in the policy. This would include monitoring by the business unit using the AI in their processes, the compliance team that is overseeing the business unit, and the audit team during periodic business audits. The use cases should only be launched when the monitoring capabilities are in place to identify unforeseen risks across all three lines of control. The primary purpose for monitoring is for the business to succeed; if interactions or decisions are being automated, the business has a value at risk and needs this monitoring to manage these processes. Monitoring for regulatory purposes is an extension of this business monitoring.
Expertise development
This brings us to expertise. AI is a new, evolving discipline that is at the first stage of mass commercialization. Hence, we believe most jobs will require additional training during this journey. Product teams need to identify problems within their domains where AI will be best leveraged. Operational teams need to execute the processes incorporating AI flawlessly, and through chaotic execution environments. Product finance teams need to be able to measure the marginal value of such incorporation into customer events. The recruiting practices need to evolve to ensure that scarce resources can balance the potential of AI with the complexity of running an intensely regulated business.
Conclusion
The incorporation of AI into processes to generate shareholder value is a once-in-a-generation change. Like all generational changes, it needs to be guided in a thoughtful and controlled way while managing risks that come along the way. Such transformations have happened before; during the early 2000s when the shift to digital posed challenges, or in the last decade as machine learning became more ingrained into core business processes. It is critical to sift the facts from the media coverage and minutia of data science. Having visibility into how AI is being used in your firm and ensuring that it is being used in accordance with the firm’s AI policy is a good start. Individual users determine the approach and potential risk, but these need to be aligned with an overall enterprise risk appetite.
We ask that you promote a culture of thoughtful decision-making, which includes taking a step back to consider all outcomes before moving forward. The principles highlighted in this paper are simple and easy to embrace and can assist the beginning of this journey. And like all things, these principles will evolve over time.
[1] Economists consider AI to be a General Purpose Technology. “A GPT has the potential to affect the entire economic system and can lead to far-reaching changes in such social factors as working hours and constraints on family life. Examples of GPTs are the steam engine, electricity, and the computer.” Elhanan Helpman, ed., General Purpose Technologies and Economic Growth (MIT Press, 1998). For application of the GPT concept to AI, see Ajay Agrawal, Joshua Gans, and Avi Goldfarb, eds., The Economics of Artificial Intelligence (University of Chicago Press, 2019). Also see the summary of the argument for AI being a GPT in Agrawal, Gans, and Goldfarb’s Power and Prediction (Harvard Business Review Press, 2022), p.14.
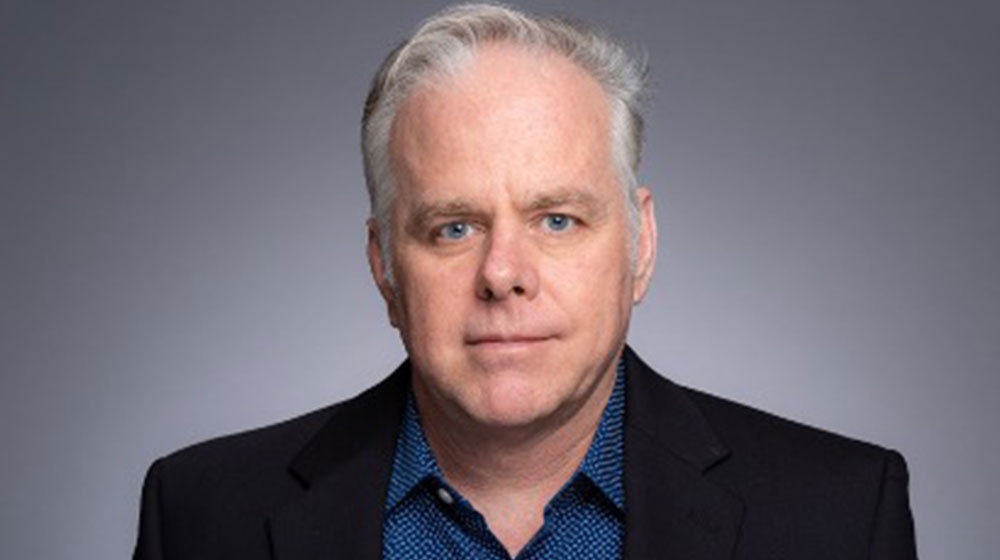
About the author
Dr. Steven Dickerson has twenty-five years of broad business experience in financial services, including serving as a Chief Analytics Officer and Chief Data Scientist. He specializes in the development, implementation, and risk management of advanced analytic products in large financial institutions. Steve earned his Ph.D. in Economics at The University of Arizona in Tucson, AZ.
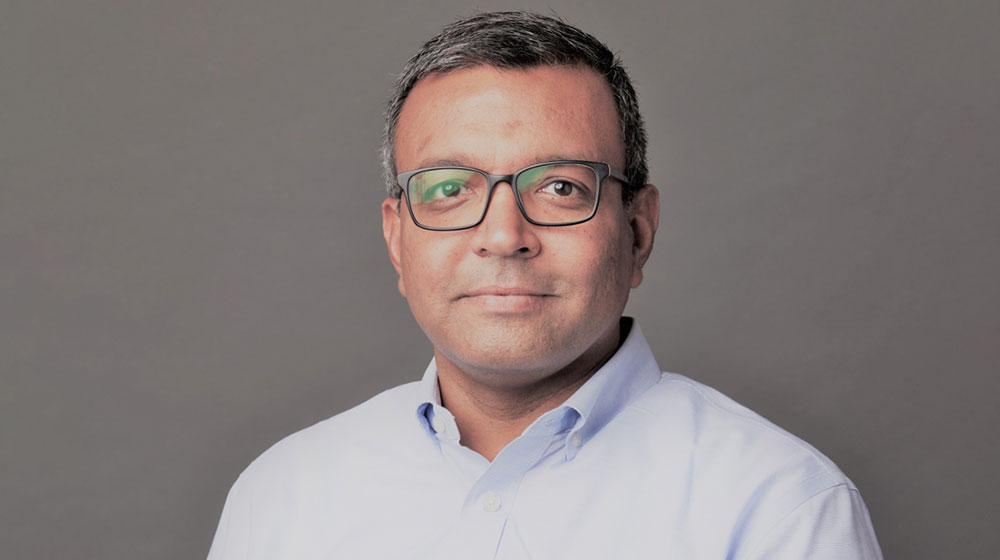
About the author
Gaurav Sharma is a seasoned Consumer Finance Executive with over 20 years of experience across credit life cycle management, digital transformation, and marketing. Most recently, as Head of Card Acquisitions Marketing at Discover Financial Services, he was responsible for and orchestrated remarkable growth for the flagship $90 Billion+ card portfolio. Prior to Discover, Gaurav worked at HSBC in India and the U.S. Gaurav has an MBA in Finance from Faculty of Management Studies, Delhi, and a Bachelor of Engineering (Honors) in Electrical Engineering from MMMUT Gorakhpur.
By Steven Dickerson, Ph.D. and Gaurav Sharma
AI is the new tool1 that will be incorporated into most processes within every firm. This journey will start with several use cases and then scale across the firm in time. The process will create both substantial value and risk. Therefore, senior leadership must ensure their organizations are ready for AI at scale. Specifically, the corporate governance structures and organizational framework of each firm must evolve to incorporate AI usage and be flexible enough to manage the changes to come. Starting with the right foundation will allow the firm to embrace AI throughout this journey. We propose a six-point checklist to assist in the initial structuring and rollout of AI:
Principle-based policy for AI use
The first step is developing a policy for your organization to follow when incorporating AI into business processes. This policy should be based on guiding principles, live within the corporate management system, have continuity with the vision and mission of the firm, and be aligned with the firm’s culture. It will provide a framework for meaningful discussions within the organization to create a path to successful integration of AI. Even if some of these principles seem out of reach at first, it is important to articulate them to clarify your firm’s aspirational focus. This is a significant change in the way teams and people work and, like all changes, will face resistance and best practices will need to be developed to support teams through this process.
The scope of the policy should reflect existing and anticipated AI usages, as well as define what will be out of bounds of the policy. The policy should also identify who “owns” the policy and define how the policy fits into the organization. We suggest including language to mitigate any disparity of approaches across the organization. This common set of principles should guide the organization throughout the lifecycle of AI: design, development, implementation, and use. Special consideration should be given to how the common set of principles applies to the acquisition and use of vendor-provided AI.
Responsible party
The policy’s zones of implementation should initially be focused on the first anticipated use cases and impact areas. It may be prudent, initially, to resist centralizing the ownership until a realistic assessment of short-term value versus long-term effort has been made. Eventually, AI needs to (1) affect a business process and (2) find its way to production for it to add value on a sustained basis. Ultimately, the risk is owned by the business, and since both of the above are business functions, the responsible party should be the business process owner. Also, the additional risk from incorporating AI into the business needs to be incorporated into the overall risk governance processes, in partnership with the risk function.
Communication
The incorporation of AI into existing processes is akin to the incorporation of customer interactions and transactions into the web in the late 1990s. There will be a lot of hyperbole, overassessment of capabilities, apprehension amongst people who think their jobs may be impacted, and underestimation of risk. The key here is to communicate candidly how you are thinking about AI, its role, the rationales for implementing it, and the first use cases. Once the use cases have been identified, seek input from subject matter experts to fine-tune the assumptions of your team to provide the best foundation for subsequent work.
Inventory
Once you have a policy that determines how AI will be integrated into your business processes, it is time to inventory existing and potential uses of AI. Using an open definition of appropriate use cases in this effort is helpful. Starting with a broader definition allows the exclusion of inventoried items rather than duplicating the effort if something is missed because of an overly narrow definition. The definition can always be refined later if it proves too broad. The inventory should include any AI developed in-house or externally, including AI embedded in a product. The inventory should capture general identification, data, methodology, and usage information for each usage. Additional questions about whether it is consistent with the guiding principles of the policy will help. The goal here is to capture the inventory as a first step.
Monitoring
Monitoring is also an essential initial step. We suggest “regularly monitored” as one of the guiding principles in the policy. This would include monitoring by the business unit using the AI in their processes, the compliance team that is overseeing the business unit, and the audit team during periodic business audits. The use cases should only be launched when the monitoring capabilities are in place to identify unforeseen risks across all three lines of control. The primary purpose for monitoring is for the business to succeed; if interactions or decisions are being automated, the business has a value at risk and needs this monitoring to manage these processes. Monitoring for regulatory purposes is an extension of this business monitoring.
Expertise development
This brings us to expertise. AI is a new, evolving discipline that is at the first stage of mass commercialization. Hence, we believe most jobs will require additional training during this journey. Product teams need to identify problems within their domains where AI will be best leveraged. Operational teams need to execute the processes incorporating AI flawlessly, and through chaotic execution environments. Product finance teams need to be able to measure the marginal value of such incorporation into customer events. The recruiting practices need to evolve to ensure that scarce resources can balance the potential of AI with the complexity of running an intensely regulated business.
Conclusion
The incorporation of AI into processes to generate shareholder value is a once-in-a-generation change. Like all generational changes, it needs to be guided in a thoughtful and controlled way while managing risks that come along the way. Such transformations have happened before; during the early 2000s when the shift to digital posed challenges, or in the last decade as machine learning became more ingrained into core business processes. It is critical to sift the facts from the media coverage and minutia of data science. Having visibility into how AI is being used in your firm and ensuring that it is being used in accordance with the firm’s AI policy is a good start. Individual users determine the approach and potential risk, but these need to be aligned with an overall enterprise risk appetite.
We ask that you promote a culture of thoughtful decision-making, which includes taking a step back to consider all outcomes before moving forward. The principles highlighted in this paper are simple and easy to embrace and can assist the beginning of this journey. And like all things, these principles will evolve over time.
[1] Economists consider AI to be a General Purpose Technology. “A GPT has the potential to affect the entire economic system and can lead to far-reaching changes in such social factors as working hours and constraints on family life. Examples of GPTs are the steam engine, electricity, and the computer.” Elhanan Helpman, ed., General Purpose Technologies and Economic Growth (MIT Press, 1998). For application of the GPT concept to AI, see Ajay Agrawal, Joshua Gans, and Avi Goldfarb, eds., The Economics of Artificial Intelligence (University of Chicago Press, 2019). Also see the summary of the argument for AI being a GPT in Agrawal, Gans, and Goldfarb’s Power and Prediction (Harvard Business Review Press, 2022), p.14.